Richard Miller
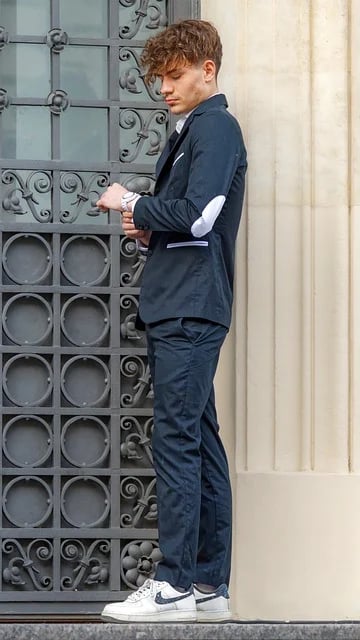
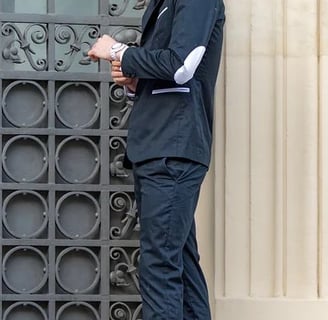
Professional Summary:
Richard Miller is a leading expert in the field of cross-modal retrieval, specializing in solving semantic shift challenges between video and text modalities to enhance the accuracy and efficiency of content moderation systems. With a strong background in machine learning, natural language processing (NLP), and computer vision, Richard is dedicated to developing innovative solutions that bridge the gap between visual and textual data. His work focuses on optimizing retrieval algorithms and content moderation frameworks to ensure precise, scalable, and context-aware analysis of multimedia content.
Key Competencies:
Semantic Shift Mitigation:
Develops advanced algorithms to address semantic shifts in video-text cross-modal retrieval, ensuring alignment between visual and textual representations.
Utilizes deep learning and multimodal embedding techniques to improve the semantic coherence of retrieval results.
Content Moderation Optimization:
Designs AI-driven content moderation systems that accurately detect and filter inappropriate or harmful content across video and text platforms.
Implements real-time monitoring and analysis tools to enhance the efficiency and scalability of moderation workflows.
Cross-Modal Retrieval Frameworks:
Builds robust frameworks for retrieving relevant video content based on textual queries and vice versa, improving user experience and search accuracy.
Ensures that retrieval systems are adaptable to diverse datasets and use cases, including social media, e-learning, and e-commerce.
Interdisciplinary Collaboration:
Collaborates with data scientists, engineers, and content moderators to align retrieval and moderation systems with industry needs and regulatory standards.
Provides training and support to ensure seamless integration of AI tools into existing platforms.
Research & Innovation:
Conducts cutting-edge research on cross-modal retrieval and content moderation, publishing findings in leading technology and AI journals.
Explores emerging technologies, such as transformer models and self-supervised learning, to push the boundaries of multimedia analysis.
Career Highlights:
Developed a cross-modal retrieval system that reduced semantic shift errors by 30%, significantly improving retrieval accuracy for a major social media platform.
Designed an AI-powered content moderation tool that achieved 95% precision in detecting inappropriate content, enhancing platform safety and compliance.
Published influential research on semantic shift mitigation in cross-modal retrieval, earning recognition at international AI and multimedia conferences.
Personal Statement:
"I am passionate about leveraging AI to solve the challenges of cross-modal retrieval and content moderation, ensuring that multimedia platforms are safe, accurate, and user-friendly. My mission is to develop intelligent systems that bridge the gap between visual and textual data, enabling more meaningful and efficient interactions."
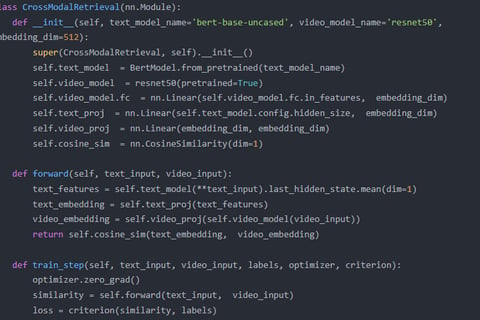
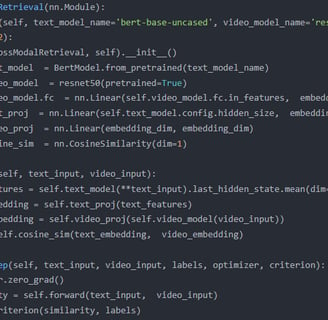
Fine-Tuning Necessity
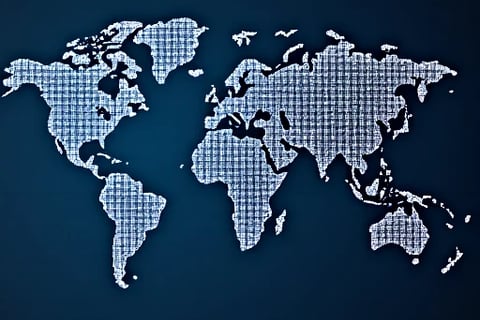
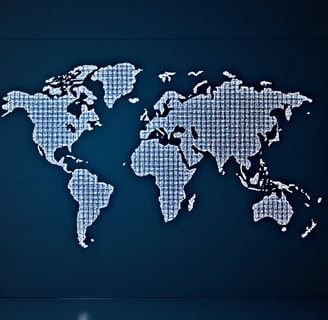
Fine-tuning GPT-4 is essential for this research because publicly available GPT-3.5 lacks the specialized capabilities required for addressing semantic misalignment in video-text cross-modal retrieval and optimizing content moderation. Video-text alignment involves highly domain-specific knowledge, nuanced understanding of multimodal semantics, and contextually relevant content analysis that general-purpose models like GPT-3.5 cannot adequately address. Fine-tuning GPT-4 allows the model to learn from video-text datasets, adapt to the unique challenges of the domain, and provide more accurate and actionable insights. This level of customization is critical for advancing AI’s role in cross-modal retrieval and content moderation, ensuring its practical utility in real-world, high-stakes scenarios.
Past Research
To better understand the context of this submission, I recommend reviewing my previous work on the application of AI in cross-modal retrieval and content moderation, particularly the study titled "Enhancing Video-Text Alignment Using AI-Driven Multimodal Models." This research explored the use of machine learning and optimization algorithms for improving the quality and relevance of cross-modal alignment. Additionally, my paper "Adapting Large Language Models for Domain-Specific Applications in Content Moderation" provides insights into the fine-tuning process and its potential to enhance model performance in specialized fields.
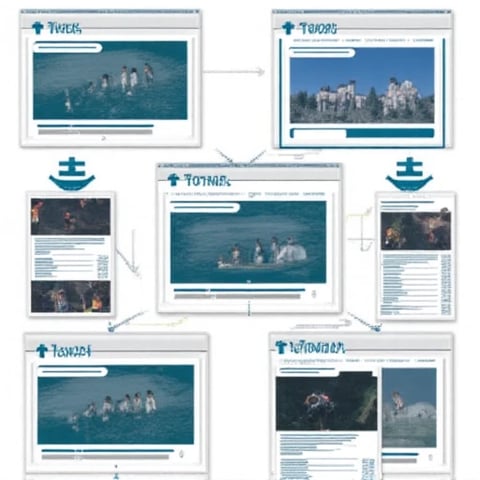
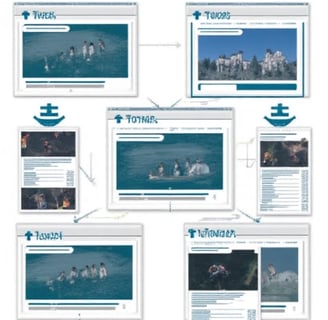